Azra AlınayT., Nida Dereli ÇalışkanE.
Metabolic engineering, initially introduced to the literature by Jay Bailey in 1991, is defined as the enhancement of cellular activities through the manipulation of enzymatic, transport, and regulatory functions of the cell using recombinant DNA technology1. Metabolic engineering, falling within the scope of industrial sectors, involves interventions in the metabolic pathways of host cells to produce targeted chemicals, fuels, pharmaceuticals, and other intermediate and end products2. With this technology, genes can be promoted and removed, and even new gene regions can be added. In order for genetic modifications to take place, suitable modification regions within the genetic materials of bacteria, which will serve as host cells, are determined. Additionally, the impact of these modifications on growth is also being investigated3.
Overview of Metabolic Engineering
Within metabolic pathways, specific biochemical processes occur. Metabolic networks are created by diagramming every metabolic pathway within an observed metabolic process. Since the target product is produced as a result of a specific pathway within the metabolic network, it is necessary to analyze the entirety of the metabolic network, including the required pathway and other pathways linked to it, in order to decipher the metabolic network4. In Figure 1, an example of the glucose metabolic network of the host cell Escherichia coli bacterium is depicted5.A fundamental mathematical approach called the stoichiometric matrix (S-matrix) method is employed To correlate metabolic steps, utilizing a table of stoichiometric coefficients for all metabolic reactions6. Once the metabolic network is constructed, modifications made on this network can be simulated to predict the new state of cellular metabolism at equilibrium using flux balance analysis (FBA)7. In metabolic engineering, when creating a specific phenotype, such as increased production of a target product (e.g., glycerol), the associated metabolic network may contain multiple metabolic pathways, and 13C-Metabolic Flux Analysis (13C-MFA) is one of the methods employed to determine the most efficient pathway. This method is more commonly utilized compared to other flux analyses due to its capability to provide more precise values for flux through its isotopic tracking mechanism and its comprehensive representation of pathway flux analysis on a broader map by targeting carbon (C), a prevalent atom in metabolic pathways8.
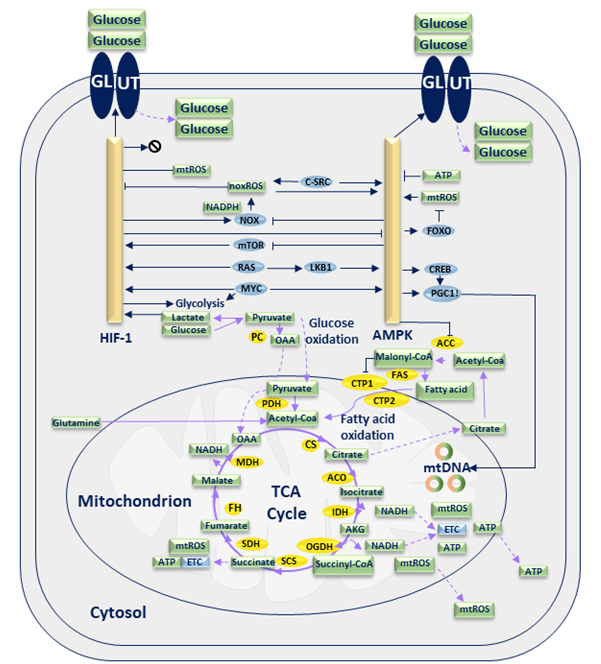
Figure 1. Glucose metabolic network of E. coli bacterium5. Steps with open arrowheads represent the products formed from the substrate, while steps with closed arrowheads indicate the substances inhibited by the substrate.
The 13C-MFA method tracks metabolites generated through metabolic processes within the cell from a labeled main substrate containing the stable isotope 13C by monitoring the isotopic quantities within these metabolites. This observation leads to obtaining realistic stoichiometric data. A realistic stoichiometric dataset for a metabolic network can be obtained using the computed and metabolic flux data. The calculated metabolic flux quantities can be depicted on the stoichiometrically curated metabolic network map either by numerical values or arrow thickness. The thickness of the arrows in the map is directly proportional to the metabolic flux of the reaction, as depicted by the flux of reaction value9. The process of integrating isotope labeling and metabolic model data into these maps is currently carried out by software tools such as METRAN10, INCA11, OpenFLUX212, and FluxML13. Differences exist between FBA and MFA methods. FBA is a mathematical approach that provides the flux dynamics at steady-state for various linear problems, including growth rate and optimization. MFA calculates the flow of the metabolic network. A comparison of these two methods is illustrated in Figure 214.

Figure 2. Comparative diagram of the applied MFA and FBA methods on the metabolic model14. Here, S represents the stoichiometric matrix, v symbolizes the flux vector, and r signifies the external metabolic rate. Calculated flux values are indicated on the arrows within the metabolic flux map.
Metabolic Engineering Today
Metabolic engineering has not only found applications in the production of foods like cheese and wine but has also shifted its focus toward pharmaceutical manufacturing15. Various steps are involved in the utilization of microorganisms for drug production:
1. Once the desired drug is chosen for production, a host cell capable of harboring the metabolic network for the drug’s precursor is selected.
2. Computational analyses performed on the chosen host cell and insights gathered from omics data (comprehensive data collected using technological and bioinformatics tools to represent biological concepts) aid in designing the experimental blueprint.
3. Through metabolic engineering, genetic modifications are applied and optimized for the required metabolic pathway.
4. The designed new cell undergoes fermentation and downstream processes to produce and isolate the product.
5. The isolated drug can be processed at an industrial scale and introduced to the market. This method facilitates the easier and cost-effective production of drugs that are otherwise difficult or expensive to manufacture (Figure 3)16.
Metabolic engineering has paved the way for drug production not only for antibiotics but also for various diseases like Alzheimer’s Disease (AD) and cancer17,18.

Figure 3. Schematic representation of the stages undergone by the target drug until it reaches the industrial-scale production phase16. Computational analysis using various bioinformatics tools is employed for drug production planning, and omics data is acquired. As a result, a plan for metabolic engineering is devised, leading to the creation of a genetically modified strain for drug production.
2-Phenethyl alcohol (Phenethyl Alcohol)
2-Phenethyl alcohol, obtainable from Bacillus licheniformis, is used in various applications due to its antibacterial and antifungal properties. It is included in the formulation of disinfectants due to these attributes19, serves as a preservative in certain medications20, and is used as an aromatic essence in perfumes due to its rose-like scent21. In a conducted study, the E. coli bacterium was chosen as the source cell to provide the necessary plasmids for genetic modifications, while B. licheniformis was selected as the host cell where genetic modifications were performed, and the production of phenethyl alcohol took place. As a result of the study, modifications made to the phenylpyruvate metabolic pathway of B. licheniformis led to an increased yield of phenethyl alcohol from glucose, reaching 6.24 g/L. The desired metabolic pathway was achieved through gene deletion using the CRISPR/Cas9 (clustered regularly interspaced palindromic repeats/CRISPR-associated protein 9 nickases) method22.
Butyrate
Histone deacetylase enzyme (HDAC) functions by removing acetyl groups from histone proteins, thereby enhancing the interaction between histones and chromatin. This action results in chromatin compaction, the formation of heterochromatin structure, and the suppression of gene expression. Histone deacetylase inhibitors (HDACi), on the other hand, reduce chromatin-histone interactions, halt the cell cycle, and promote apoptosis and autophagy23. In addition to these functions, they also exhibit anti-cancer effects by activating tumor-suppressor genes24. One such HDAC inhibitor (HDA-Ci) is butyrate, which is a cancer-fighting compound found particularly in colon cancer treatments25. In a study, this compound was heterologously expressed in Bacteroides thetaiotaomicron bacteria, meaning it was transferred to another plasmid to activate the butyrate biosynthesis pathway. As a result, butyrate production was achieved at a quantity of 12mg/L18.
Biopterin
Biopterin is a compound involved in the production of neurotransmitters such as dopamine, serotonin, adrenaline, and noradrenaline. It can be utilized for treating neurotransmitter deficiencies26. The production of biopterin can be achieved through heterologous expression of the biopterin biosynthesis pathway in Pseudomonas putida bacteria using the CRISPR method27.
Cyclo-inositol
It is believed that one of the causes of Alzheimer’s disease (AD) is the accumulation of plaques around neurons due to misfolding of amyloid beta (Aß) proteins. The compound cyclo-inositol is utilized as a drug active ingredient in the treatment of AD due to its ability to inhibit toxic Aß proteins28. Cyclo-inositol can be obtained from the bacterium Corynebacterium glutamicum. Through various genetic modifications including oxiC-cg3390-oxiD-oxiE gene deletions and other deletion and insertion motifs, myo-inositol production was supported in C. glutamicum bacteria. Subsequently, the production of the cyclo-inositol molecule was achieved from myo-inositol28. As a result of the conducted study, a solution containing 4.4 g/L of cyclo-inositol was obtained from a 20 g/L glucose solution17.
Violacein
The compound violacein is used as an anti-tumor agent due to its ability to inhibit Death- associated protein kinase 1 (DAPK1), which prevents apoptosis, and to block cell survival signals29. Manipulation of the vioD gene expressed in the tryptophan pathway of E. coli bacteria enables the production of violacein from tryptophan30.
Paclitaxel (Taxol)
Cell division during the cell cycle is facilitated by the movements of microtubules formed through the heterodimerization of alpha (α)- and beta (β)-tubulin monomers31. The compound paclitaxel inhibits the polymerization of these subunits, thereby preventing cell division. Due to this function, it can be used as an anti-cancer agent in chemotherapy treatment32. In the last five years, metabolic engineering of paclitaxel production in different host cells has focused on enhancing the expression of pathway elements during the production stages. This includes catalysts such as taxadiene 5-alpha hydroxylase (T5αH)33-35, taxadiene synthase (TXS)34,36,37, and deacetyl baccatin III-10-β-O-acetyltransferase (DBAT)38-40, and various studies have been conducted to achieve this goal. As a result of these efforts, ongoing metabolic engineering studies aim to achieve more cost-effective production of paclitaxel, typically obtained from the Taxus brevifolia plant, than bacterial systems41.
In conclusion, metabolic engineering is an approach aimed at the production of necessary compounds for biofuel, chemical, and pharmaceutical manufacturing through genetic modifications in a host cell and interventions in the metabolic network of that cell. In this approach, which is created through the collaboration of engineering and biological sciences, the fundamental requirement is to detect the host cell’s metabolism. For this purpose, metabolic networks and metabolic pathways within the network are investigated. In order to obtain a realistic metabolic network, quantitative analyses of the investigated metabolism and mapping of the stoichiometric data of reactions onto the metabolic network are necessary. For this purpose, a mathematical approach known as the S-matrix, a matrix containing the ratios of products to substrates in metabolic reactions within the network, is used.
FBA is a stoichiometric matrix-based mathematical approach that provides the solution to the steady-state flux distribution of linear problems, including growth rate, optimization, and more. Additionally, 13C–MFA allows the calculation of metabolic fluxes through the metabolic network, enabling the acquisition of realistic information about flux distribution. Software tools such as Metran, INCA, OpenFLUX, and FluxML are utilized for MFA. In current times, metabolic engineering enables the production of substances like 2-phenylethanol, butyrate, biopterin, cyclo-inositol, violacein, and paclitaxel through bacterial systems. It is believed that metabolic engineering could lead to easier and more cost-effective drug production in the future, potentially enhancing patient access to medication and consequently improving healthcare outcomes. Moreover, it has the potential to increase the production of molecules necessary for scientific experiments, accelerating scientific advancements. Additionally, it might even contribute to unforeseen developments, fostering progress in various fields beyond current predictions.
References:
- Bailey, J. E. (1991). Toward a Science of Metabolic Engineering. Science, 252(5013), 1668–1675. https://doi.org/10.1126/science.2047876
- Hande Tekarslan-Sahin, S. (2021). Metabolic Engineering of Saccharomyces cerevisiae for Industrial Biotechnology. In T. P. Basso & L. C. Basso (Eds.), Saccharomyces (p. 1). https://doi.org/10.5772/intechopen.96030
- Yadav, D., Tanveer, A., Malviya, N., & Yadav, S. (2018). Overview and principles of bioengineering: The drivers of omics technologies. In Omics Technologies and Bio-engineering: Towards Improving Quality of Life (Vol. 1, pp. 3–23). Elsevier Inc. https://doi.org/10.1016/B978-0-12-804659-3.00001-4
- Dräger, A., & Planatscher, H. (2013). Metabolic Networks. In W. Dubitzky, O. Wolkenhauer, K. Cho, & H. Yokota (Eds.), Encyclopedia of Systems Biology. Springer. https://doi.org/10.1007/978-1-4419-9863-7_1277
- Jia, D., Lu, M., Jung, K. H., Park, J. H., Yu, L., Onuchic, J. N., Kaipparettu, B. A., & Levine, H. (2019). Elucidating cancer metabolic plasticity by coupling gene regulation with metabolic pathways. Proceedings of the National Academy of Sciences of the United States of America, 116(9), 3909–3918. https://doi.org/10.1073/pnas.1816391116
- Resendis-Antonio, O. (2013). Stoichiometric Matrix. In Encyclopedia of Systems Biology (p. 2014). Springer. https://doi.org/10.1007/978-1-4419-9863-7_1366
- Orth, J. D., Thiele, I., & Palsson, B. O. (2010). What is flux balance analysis? In Nature Biotechnology (Vol. 28, Issue 3, pp. 245–248). https://doi.org/10.1038/nbt.1614
- Long, C. P., & Antoniewicz, M. R. (2019). High-resolution 13C metabolic flux analysis. Nature Protocols, 14(10), 2856–2877. https://doi.org/10.1038/s41596-019-0204-0
- Foguet, C., Jayaraman, A., Marin, S., Selivanov, V. A., Moreno, P., Messeguer, R., de Atauri, P., & Cascante, M. (2019). P13CMFA: Parsimonious 13C metabolic flux analysis. PLoS Computational Biology, 15(9). https://doi.org/10.1371/journal.pcbi.1007310
- Yoo, H., Antoniewicz, M. R., Stephanopoulos, G., & Kelleher, J. K. (2008). Quantifying reductive carboxylation flux of glutamine to lipid in a brown adipocyte cell line. Journal of Biological Chemistry, 283(30), 20621–20627. https://doi.org/10.1074/jbc.M706494200
- Rahim, M., Ragavan, M., Deja, S., Merritt, M. E., Burgess, S. C., & Young, J. D. (2022). INCA 2.0: A tool for integrated, dynamic modeling of NMR- and MS-based isotopomer measurements and rigorous metabolic flux analysis. Metabolic Engineering, 69, 275–285. https://doi.org/10.1016/j.ymben.2021.12.009
- Shupletsov, M. S., Golubeva, L. I., Rubina, S. S., Podvyaznikov, D. A., Iwatani, S., & Mashko, S. v. (2014). OpenFLUX2: 13C-MFA modeling software package adjusted for the comprehensive analysis of single and parallel labeling experiments. Microbial Cell Factories, 13(1). https://doi.org/10.1186/s12934-014-0152-x
- Keld Nielsen, L., Cortassa, S., Shimizu, H., Antoniewicz, M. R., Nöh, K., Beyß, M., Azzouzi, S., Weitzel, M., & Wiechert, W. (2019). The Design of FluxML: A Universal Modeling Language for 13 C Metabolic Flux Analysis. Frontiers in Microbiology | Frontiersin, 1, 1022. https://doi.org/10.3389/fmicb.2019.01022
- Emwas, A. H., Szczepski, K., Al-Younis, I., Lachowicz, J. I., & Jaremko, M. (2022). Fluxomics – New Metabolomics Approaches to Monitor Metabolic Pathways. In Frontiers in Pharmacology (Vol. 13). Frontiers Media S.A. https://doi.org/10.3389/fphar.2022.805782
- Mallikarjuna, N., & Yellamma, K. (2018). Genetic and metabolic engineering of microorganisms for the production of various food products. In Recent Developments in Applied Microbiology and Biochemistry (pp. 167–182). Elsevier. https://doi.org/10.1016/B978-0-12-816328-3.00013-1
- Lee, S. Y., Kim, H. U., Park, J. H., Park, J. M., & Kim, T. Y. (2009). Metabolic engineering of microorganisms: general strategies and drug production. In Drug Discovery Today (Vol. 14, Issues 1–2, pp. 78–88). https://doi.org/10.1016/j.drudis.2008.08.004
- Ramp, P., Lehnert, A., Matamouros, S., Wirtz, A., Baumgart, M., & Bott, M. (2021). Metabolic engineering of Corynebacterium glutamicum for production of scyllo-inositol, a drug candidate against Alzheimer’s disease. Metabolic Engineering, 67, 173–185. https://doi.org/10.1016/j.ymben.2021.06.011
- Kim, K., Choe, D., Song, Y., Kang, M., Lee, S. G., Lee, D. H., & Cho, B. K. (2021). Engineering Bacteroides thetaiotaomicron to produce non-native butyrate based on a genome-scale metabolic model-guided design. Metabolic Engineering, 68, 174–186. https://doi.org/10.1016/j.ymben.2021.10.005
- Martínez-Avila, O., Sánchez, A., Font, X., & Barrena, R. (2018). Bioprocesses for 2-phenylethanol and 2-phenylethyl acetate production: current state and perspectives. In Applied Microbiology and Biotechnology (Vol. 102, Issue 23, pp. 9991–10004). Springer Verlag. https://doi.org/10.1007/s00253-018-9384-8
- Reza, H., Fereshteh, N., & Saman, A. N. (2015). Determination of phenylethyl alcohol by reversed-phase high-performance liquid chromatography (RP-HPLC) in Budesonide nasal spray. African Journal of Pure and Applied Chemistry, 9(5), 81–90. https://doi.org/10.5897/ajpac2015.0619
- Chantasuban, T., Santomauro, F., Gore-Lloyd, D., Parsons, S., Henk, D., Scott, R. J., & Chuck, C. (2018). Elevated production of the aromatic fragrance molecule, 2-phenylethanol, using Metschnikowia pulcherrima through both de novo and ex novo conversion in batch and continuous modes. https://doi.org/10.1002/jctb.5597
- Zhan, Y., Shi, J., Xiao, Y., Zhou, F., Wang, H., Xu, H., Li, Z., Yang, S., Cai, D., & Chen, S. (2022). Multilevel metabolic engineering of Bacillus licheniformis for de novo biosynthesis of 2-phenylethanol. Metabolic Engineering, 70, 43–54. https://doi.org/10.1016/j.ymben.2022.01.007
- Soares, C. P., Brancolini, C., Naples, L. V., Lu, I. X., Zhu, W.-G., Li, G., & Tian, Y. (2020). The Roles of Histone Deacetylases and Their Inhibitors in Cancer Therapy. https://doi.org/10.3389/fcell.2020.576946
- Eckschlager, T., Plch, J., Stiborova, M., & Hrabeta, J. (2017). Histone deacetylase inhibitors as anticancer drugs. In International Journal of Molecular Sciences (Vol. 18, Issue 7). MDPI AG. https://doi.org/10.3390/ijms18071414
- Pradhan, N., Kar, S., Parbin, S., Sengupta, D., Deb, M., Das, L., & Patra, S. K. (2019). Epigenetic Dietary Interventions for Prevention of Cancer. Epigenetics of Cancer Prevention, 23–48. https://doi.org/10.1016/B978-0-12-812494-9.00002-0
- Ohashi, A., Saeki, Y., Harada, T., Naito, M., Takahashi, T., Aizawa, S., & Hasegawa, H. (2016). Tetrahydrobiopterin supplementation: Elevation of tissue biopterin levels accompanied by a relative increase in dihydrobiopterin in the blood and the role of probenecid-sensitiveuptake in scavenging dihydrobiopterin in the liver and kidney of rats. PLoS ONE, 11(10). https://doi.org/10.1371/journal.pone.0164305
- Kiattisewee, C., Dong, C., Fontana, J., Sugianto, W., Peralta-Yahya, P., Carothers, J. M., & Zalatan, J. G. (2021). Portable bacterial CRISPR transcriptional activation enables metabolic engineering in Pseudomonas putida. Metabolic Engineering, 66, 283–295. https://doi.org/10.1016/j.ymben.2021.04.002
- Lee, D., Lee, W.-S., Lim, S., Kim, Y. K., Jung, H.-Y., Das, S., Lee, J., Luo, W., Kim, K.-T., & Chung, S.-K. (2017). A guanidine-appended scyllo-inositol derivative AAD-66 enhances brain delivery and ameliorates Alzheimer’s phenotypes OPEN. https://doi.org/10.1038/s41598-017-14559-7
- Durán, N., Nakazato, G., Durán, M., Berti, I. R., Castro, G. R., Stanisic, D., Brocchi, M., Wagner, ·, Fávaro, J., Ferreira-Halder, C. v, Justo, G. Z., & Tasic, L. (2021). Multi-target drug with potential applications: violacein in the spotlight. World Journal of Microbiology and Biotechnology, 37, 151. https://doi.org/10.1007/s11274-021-03120-4
- Rodrigues, A. L., Trachtmann, N., Becker, J., Lohanatha, A. F., Blotenberg, J., Bolten, C. J., Korneli, C., de Souza Lima, A. O., Porto, L. M., Sprenger, G. A., & Wittmann, C. (2013). Systems metabolic engineering of Escherichia coli for production of the antitumor drugs violacein and deoxyviolacein. Metabolic Engineering, 20, 29–41. https://doi.org/10.1016/j.ymben.2013.08.004
- Binarová, P., & Tuszynski, J. (2019). Tubulin: Structure, Functions and Roles in Disease. Cells, 8(1294). https://doi.org/10.3390/cells8101294
- Weaver, B. A. (2014). How Taxol/paclitaxel kills cancer cells. 25. https://doi.org/10.1091/mbc.E14-04-0916
- Edgar, S., Zhou, K., Qiao, K., King, J. R., Simpson, J. H., & Stephanopoulos, G. (2016). Mechanistic Insights into Taxadiene Epoxidation by Taxadiene-5α-Hydroxylase. ACS Chemical Biology, 11(2), 460–469. https://doi.org/10.1021/acschembio.5b00767
- Edgar, S., Li, F. S., Qiao, K., Weng, J. K., & Stephanopoulos, G. (2017). Engineering of Taxadiene Synthase for Improved Selectivity and Yield of a Key Taxol Biosynthetic Intermediate. ACS Synthetic Biology, 6(2), 201–205. https://doi.org/10.1021/acssynbio.6b00206
- Yadav, V. G. (2014). Unraveling the multispecificity and catalytic promiscuity of taxadiene monooxygenase. Journal of Molecular Catalysis B: Enzymatic, 110, 154–164. https://doi.org/10.1016/j.molcatb.2014.10.004
- Schrepfer, P., Buettner, A., Goerner, C., Hertel, M., Rijn, J. van, Wallrapp, F., Eisenreich, W., Sieber, V., Kourist, R., & Brück, T. (2016). Identification of amino acid networks governing catalysis in the closed complex of class i terpene synthases. Proceedings of the National Academy of Sciences of the United States of America, 113(8), E958–E967. https://doi.org/10.1073/pnas.1519680113
- Ansbacher, T., Freud, Y., & Major, D. T. (2018). Slow-Starter Enzymes: Role of Active-Site Architecture in the Catalytic Control of the Biosynthesis of Taxadiene by Taxadiene Synthase. Biochemistry, 57(26), 3773–3779. https://doi.org/10.1021/acs.biochem.8b00452
- Li, B. J., Wang, H., Gong, T., Chen, J. J., Chen, T. J., Yang, J. L., & Zhu, P. (2017). Improving 10-deacetylbaccatin III-10-β-O-acetyltransferase catalytic fitness for Taxol production. Nature Communications, 8. https://doi.org/10.1038/ncomms15544
- You, L. F., Huang, J. J., Wei, T., Lin, S. L., Jiang, B. H., Guo, L. Q., & Lin, J. F. (2018). Enhanced catalytic activities and modified substrate preferences for taxoid 10β-O-acetyl transferase mutants by engineering catalytic histidine residues. Biotechnology Letters, 40(8), 1245–1251. https://doi.org/10.1007/s10529-018-2573-9
- Lin, S. L., Wei, T., Lin, J. F., Guo, L. Q., Wu, G. P., Wei, J. bin, Huang, J. J., & Ouyang, P. L. (2018). Bio-production of Baccatin III, an Important Precursor of Paclitaxel by a Cost-Effective Approach. Molecular Biotechnology, 60(7), 492–505. https://doi.org/10.1007/s12033-018-0090-7
Mutanda, I., Li, J., Xu, F., & Wang, Y. (2021). - Recent Advances in Metabolic Engineering, Protein Engineering, and Transcriptome-Guided Insights Toward Synthetic Production of Taxol. In Frontiers in Bioengineering and Biotechnology (Vol. 9). Frontiers Media S.A. https://doi.org/10.3389/fbioe.2021.632269